Smart Ways to Optimize Degrees of Freedom in Statistical Analysis
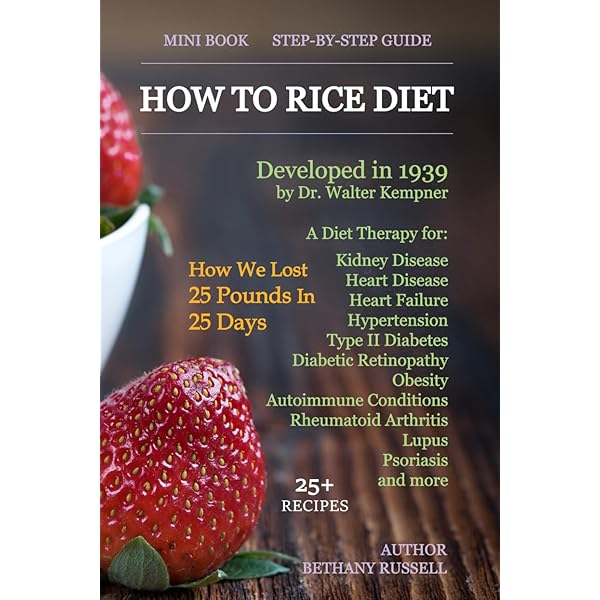
Effective Ways to Find Degrees of Freedom in Statistical Analysis
The concept of degrees of freedom plays a crucial role in various statistical analyses, influencing the validity of results in research methodology. Understanding how to find and interpret degrees of freedom can enhance the power of statistical tests such as the t-test, ANOVA, and chi-square tests. In this article, we will delve into effective methods for calculating degrees of freedom within the context of statistical analysis.
The Definition and Importance of Degrees of Freedom
The term degrees of freedom refers to the number of independent data values that can vary in a statistical calculation. This concept is vital because it helps determine the critical values used in hypothesis testing, shaping conclusions drawn from statistical inference. For example, in a t-distribution, the degrees of freedom are derived from the sample size; specifically, it is calculated as the sample size minus one. This adjustment accounts for the estimation of the population mean, resulting in more accurate confidence intervals and significance tests.
Calculating Degrees of Freedom for Different Tests
Different statistical tests require distinct approaches for calculating degrees of freedom. In the case of the t-test, the formula for degrees of freedom is simply the number of samples minus one (n - 1), where 'n' is the sample size. During regression analysis, the degrees of freedom can be more complex, often calculated as the difference between the number of observations and the number of estimated parameters. For instance, a simple linear regression involving one predictor variable would have degrees of freedom equal to (n - 2), since one degree is used to estimate the slope and another for the intercept. Understanding these formulas is crucial for proper application in research settings.
Types of Degrees of Freedom in Analysis
Degrees of freedom can be categorized based on the type of analysis being conducted. In ANOVA (Analysis of Variance), degrees of freedom are divided into two types: between-group and within-group degrees of freedom. The between-group degree of freedom is computed as the number of groups minus one (k - 1), while the within-group is calculated as the total number of observations minus the number of groups (n - k). This differentiation helps researchers understand the sources of variability in their data sets and allows for more informed conclusions.
Calculating Degrees of Freedom: Practical Examples
Practical examples help clarify the concept of degrees of freedom. For instance, if a researcher conducts a t-test with a sample size of 30 individuals to investigate the impact of a new medication, they would calculate degrees of freedom as 30 - 1 = 29. Understanding these calculations is essential in setting correct significance levels and avoiding erroneous conclusions. In the context of chi-square tests, the degree of freedom is determined based on the formula (rows - 1) × (columns - 1). For example, a 2x2 contingency table would yield (2 - 1)(2 - 1) = 1 degree of freedom.
Examples of Degrees of Freedom in Regression
In regression analysis, recognizing degrees of freedom can illustrate the model's complexity and performance. Suppose you are conducting a multiple regression analysis with 5 predictors. The total degrees of freedom would be calculated from the data points available. If there are 100 observations, the degrees of freedom associated with the regression would be 100 - 5 - 1 = 94. This reflects the number of independent observations available for estimating the precision of our predicted model outcomes.
Exploring Degrees of Freedom in ANOVA
When performing an ANOVA, seeing the impact of degree calculations amplifies the analysis. For example, in a study assessing the impact of three different teaching methods on student performance across 30 participants, the between-group degrees of freedom would be calculated as (3 - 1) = 2, while the within-group degrees of freedom would thus be (30 - 3) = 27, leading to a total of (30 - 1) = 29 degrees of freedom. This understanding lends insight into the statistical power of analyzing variance effectively.
Degrees of Freedom in Hypothesis Testing
Within the realm of hypothesis testing, understanding the implications of degrees of freedom can significantly influence research outcomes. The concept serves as a guide for how close data approximates theories or expectations under the null hypothesis. For example, in determining how the chi-square statistic translates into the context of hypothesis testing, the calculation of degrees of freedom is integral to retrieving the critical value needed for significance levels. Using a degrees of freedom chart can assist researchers in interpreting p-values correctly, ensuring reliable conclusions are drawn from empirical studies.
The Role of Degrees of Freedom in Variance Analysis
In variance analysis, recognizing how degrees of freedom interact with calculations is essential for quantifying the variability present in data. The F-statistic in ANOVA, used for comparing variances across groups, directly relies on degrees of freedom for both group and error calculations. This hierarchical understanding empowers researchers to qualify results and measures against known standards within the context of statistical relevance, thus enhancing their analysis.
Interpreting Degrees of Freedom Across Variables
Effective interpretation of degrees of freedom, especially in studies with multiple independent variables, enhances the decision-making process. Each variable added to a model reduces degrees of freedom due to increased parameter estimation requirements. For instance, focusing on how many predictors can fit into a dataset while maintaining statistical power becomes crucial in current statistical practice. Such evaluations ensure a balance between model complexity, fit, and Model performance via metrics like the Akaike Information Criterion (AIC).
Key Takeaways
- Degrees of freedom are critical for calculating statistics used in hypothesis testing.
- Different statistical methods require different calculations regarding degrees of freedom.
- Understanding degrees of freedom improves the reliability and validity of results in empirical research.
- Degrees of freedom impact the shape of sampling distributions important in significance testing.
- Inclusion of various independent variables influences degrees of freedom and hence should be carefully evaluated during analyses.
FAQ
1. What is the definition of degrees of freedom in statistics?
Degrees of freedom in statistics refers to the number of independent values or quantities that can change when calculating a statistic. It is an essential concept for understanding how sample size impacts the reliability of estimates, especially in analysis tests.
2. What are the different types of degrees of freedom?
There are several types of degrees of freedom, notably: the total degrees of freedom, which is calculated from the sample size, and the within-versus between-group degrees of freedom, used particularly in ANOVA. Specifically, they assist researchers in deciphering sources of variability within datasets.
3. How do degrees of freedom differ in regression and ANOVA?
In regression analysis, degrees of freedom emphasize the number of observations reduced by the number of parameters tested. Conversely, ANOVA separates between-group and within-group contributions to variability, each with its formulas reflecting different aspects of the dataset.
4. Why are degrees of freedom important in hypothesis testing?
Degrees of freedom are crucial in setting the significance level in hypothesis testing. They affect the probability distributions used, which ultimately helps in determining whether observed results could occur by chance under the null hypothesis.
5. How can I calculate degrees of freedom for different statistical tests?
The calculation methods depend on the test type. For t-tests, degrees of freedom are usually n - 1. In ANOVA, the calculations are k - 1 for between groups and n - k for within groups. Understanding these calculations enhances proper application in studies.
6. What is the application of degrees of freedom in regression analysis?
In regression analysis, degrees of freedom helps quantify how many independent observations remain once the parameters have been estimated. This has implications for model accuracy and predictive performance, allowing researchers to adjust models more effectively.
7. Can degrees of freedom affect the power of a statistical test?
Yes, degrees of freedom directly influence the statistical power. Greater degrees of freedom usually lead to more reliable estimates and models, enhancing the likelihood of correctly rejecting a false null hypothesis within the context of hypothesis testing.
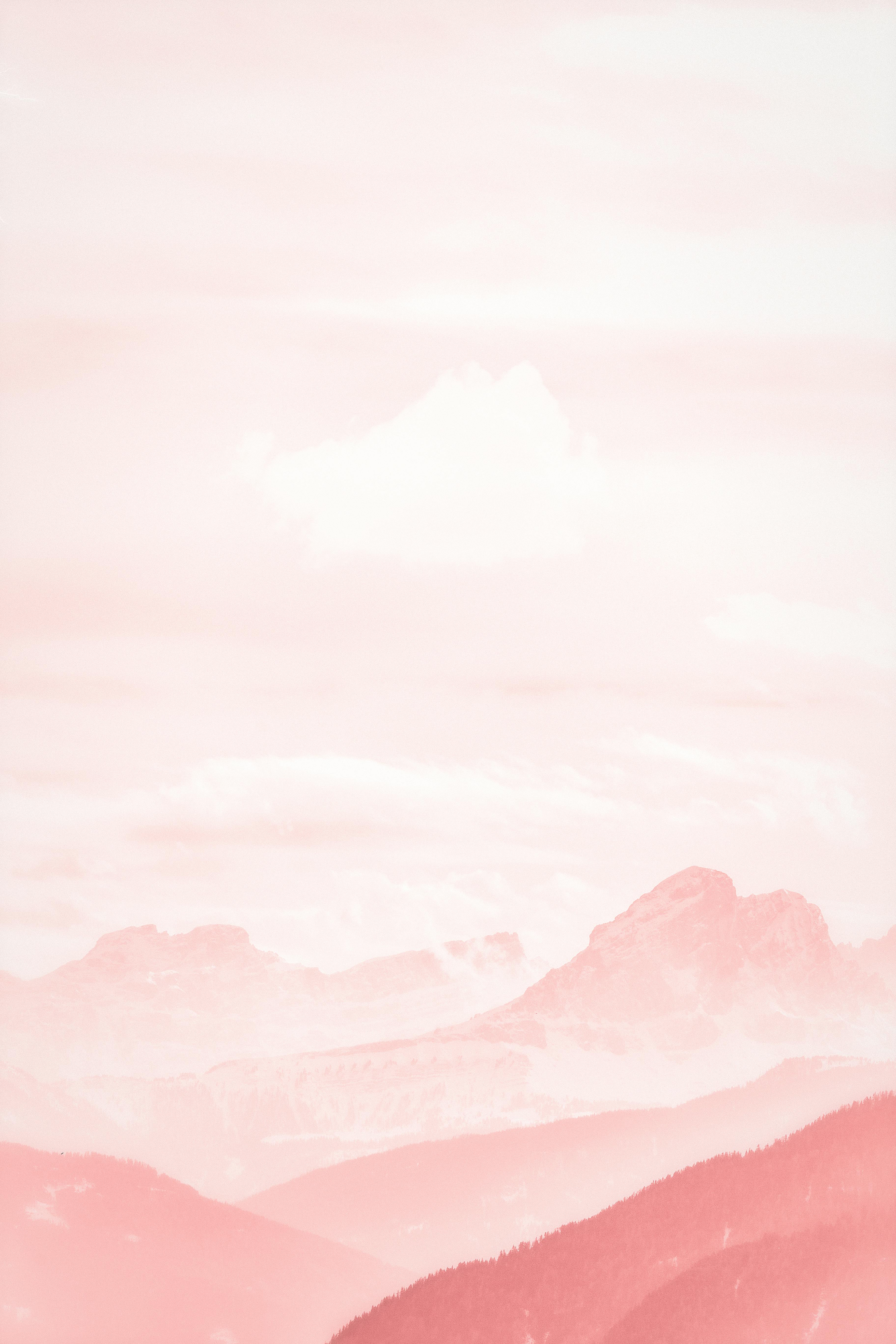
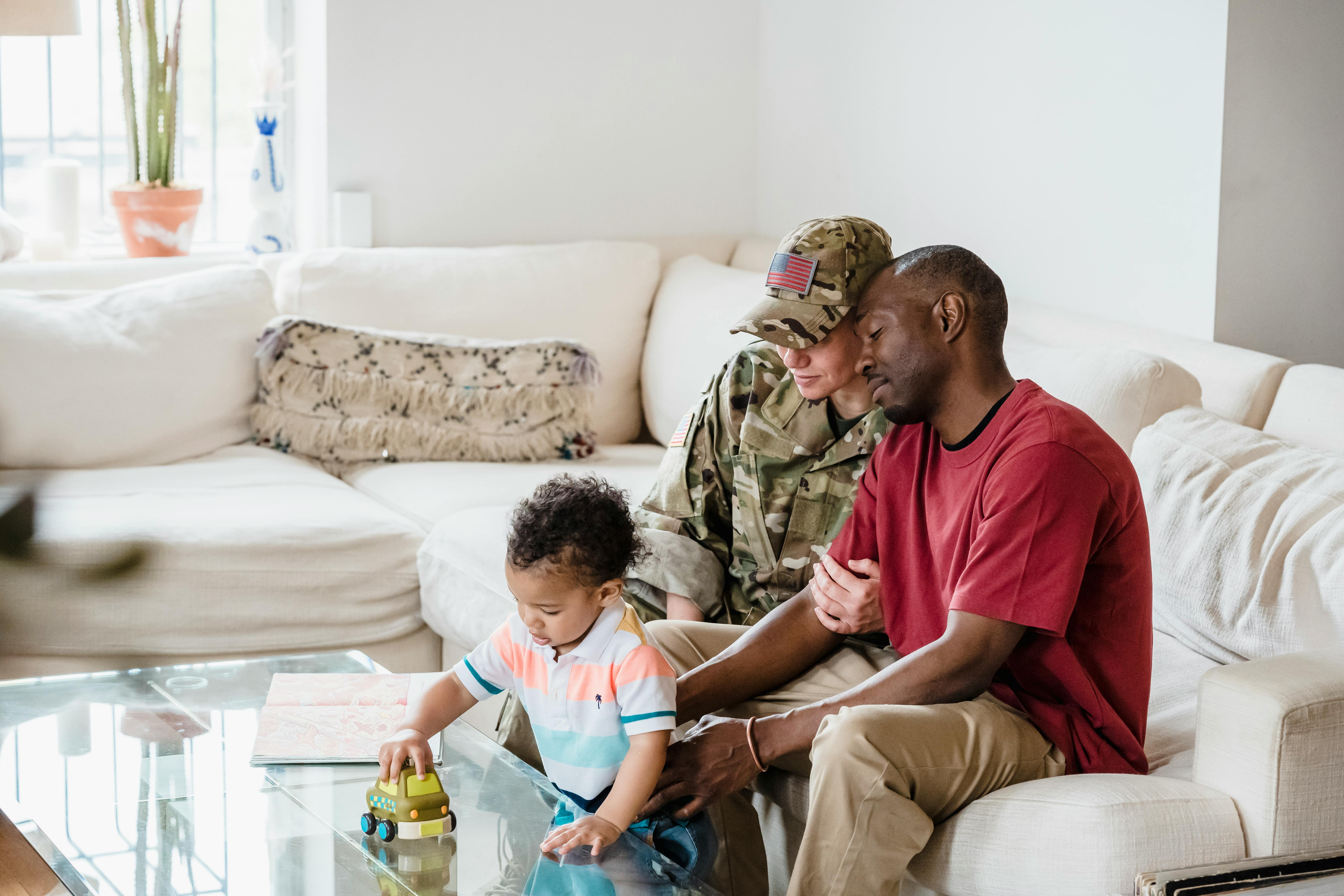