How to Discover the IQR: Effective Methods for Data Analysis in 2025
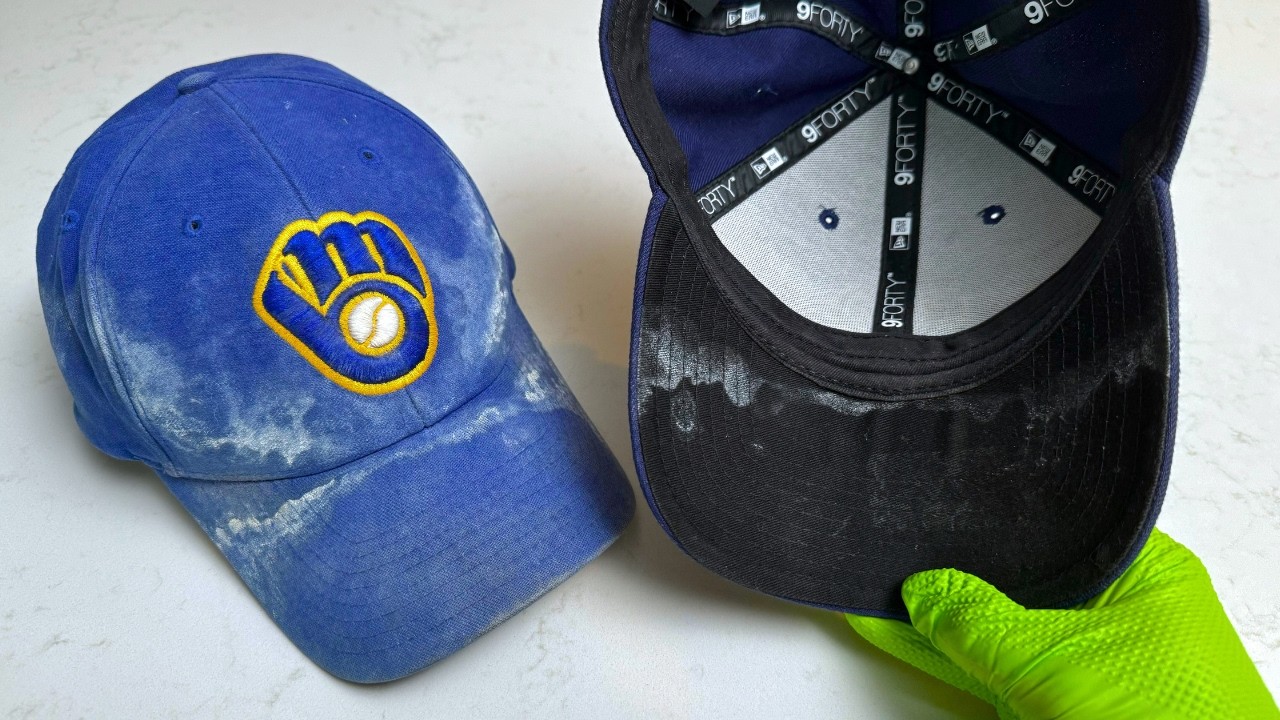
How to Find the IQR: A Practical Guide for Data Analysis in 2025
Understanding the Interquartile Range (IQR)
The interquartile range (IQR) is a vital concept in statistical analysis, serving as a robust measure of variability within data sets. It captures the middle 50% of a dataset, providing insights into the data's spread. Essentially, the IQR is the difference between the upper quartile (Q3) and the lower quartile (Q1). Understanding how to find the IQR enables data analysts to determine the presence of outliers and assess the distribution's quartiles effectively. This makes the IQR crucial for data analysis techniques, especially when visualizing data through box plots, which rely on IQR values to convey the data's significance accurately.
The IQR Formula
To establish an in-depth understanding of how to find the IQR, we must first get familiar with the IQR formula. The formula itself is simple: IQR = Q3 - Q1. Here, Q3 is the upper quartile that marks the 75th percentile, while Q1 is the lower quartile that accounts for the 25th percentile of the dataset. To compute the IQR, firstly, the quartiles must be located. For a given dataset, arrange the numbers in ascending order, then find the median as well as the Q1 and Q3 positions. Understanding this process enhances one’s capacity for effective research analysis and thorough data exploration in various contexts.
Data Representation Using Box Plots
Once we have computed the IQR, a box plot is an excellent way to visualize this information. A box plot (or box-and-whisker plot) effectively represents the IQR and helps communicate the data distribution succinctly. The box in the box plot illustrates the IQR, while the line inside the box shows the median. The 'whiskers' indicate the range of data, highlighting potential outliers that fall outside 1.5 times the IQR from either quartile. This visual representation is crucial for comparing distributions across different datasets, ultimately aiding in identifying trends and outliers efficiently. Incorporating visualizing IQR techniques into your statistical analysis can greatly enhance understanding and communication of these data insights.
In-Depth Techniques for Calculating IQR
When it comes to calculating the IQR, employing effective techniques can improve accuracy and the overall understanding of data spread analysis. From handling small sample sizes to larger data sets, various methods can be utilized to derive the quartiles and subsequently the IQR.
Step-by-Step Process for Calculating IQR
A systematic way to compute the IQR involves the following steps: First, organize your dataset in ascending order. Next, find the median, which divides the data into two halves. The lower quartile (Q1) is the median of the lower half, while the upper quartile (Q3) is the median of the upper half. Once both quartiles are identified, simply subtract Q1 from Q3 to obtain the IQR. This step-by-step approach ensures that complexity does not hinder understanding, aligning with effective data analysis methodologies.
Using Software Tools for IQR Calculation
In advanced statistical analysis, software tools play a significant role in calculating the IQR effectively. Programs like R, Python, or Excel can automate the process of quartile calculation through built-in functions, allowing users to handle larger datasets comfortably. For instance, in Python, the ‘numpy’ and ‘pandas’ libraries offer functions such as np.percentile() for quick IQR calculations, facilitating timely evaluations of IQR data. Adopting statistical tools for IQR computations not only enhances productivity but also maximizes accuracy, making it a necessary consideration for modern analysts.
Practical Examples of Using IQR in Data Analysis
Understanding how to apply the IQR in various data analysis scenarios facilitates better data interpretation and enhances statistical understanding. Here, we will cover practical examples illustrating its application across different contexts.
Identifying Outliers with IQR
One of the primary applications of IQR is in the identification of outliers. Data points that fall beyond 1.5 times the IQR from Q1 and Q3 can be classified as outliers. For example, if Q1 is 10 and Q3 is 20, the IQR would be calculated as 10. Multiplying the IQR by 1.5 gives us 15. Therefore, any data point below 10 - 15 = -5 or above 20 + 15 = 35 would be an outlier. This method provides a standardized approach to flag deviations in data groupings and facilitate a clearer understanding of data distributions, embodying unique insights into the data's nature.
Case Study: Evaluating Survey Data with IQR
Consider a scenario where a company conducts a customer satisfaction survey with ratings on a scale from 1 to 100. By calculating the IQR of the responses, the company can uncover valuable insights regarding customer experience. If Q1 is 65 and Q3 is 85, resulting in an IQR of 20, the intersection of this data range can be instrumental in understanding customer satisfaction levels comprehensively. Analyzing response patterns using the IQR helps in effective decision-making and strategic planning around service improvements.
Why is IQR Important in Statistical Analysis?
The importance of the IQR in statistical analysis extends beyond mere computation. It serves various essential functions that enhance overall data understanding.
Variability Measurement with IQR
The IQR effectively encapsulates the variability within the dataset. By focusing on the central 50%, it provides a transparent view of data spread, which is less susceptible to the influence of extreme outliers compared to other statistical measures such as range. This quality makes it a favorite among statisticians seeking to provide a reliable variability measurement in data presentations. In decision-making scenarios and data storytelling, utilizing the IQR allows for a well-rounded analytical model.
Enhancing Data Comparisons Using IQR
Analyzing multiple datasets is often required in research, and the IQR provides an effective means of comparison. By studying the IQR of different groups, analysts can quickly identify which dataset exhibits greater variability or if certain groups contrast significantly concerning variability. This capability of comparing distributions enhances research depth, creating opportunities for further investigations and analyses.
Key Takeaways
- The IQR is a vital statistical tool for analyzing data spread and identifying outliers.
- Computation involves calculating Q1 and Q3 and using the formula IQR = Q3 - Q1.
- Visualizing the IQR using box plots is effective for communicating data insights.
- Understanding how to apply IQR enhances decision-making and strategic planning.
- Statistical tools like Python and R can streamline the IQR calculation process for larger datasets.
FAQ
1. What is the basic definition of IQR?
The interquartile range (IQR) is defined as the range between the first quartile (Q1) and the third quartile (Q3) of a dataset, effectively capturing the middle 50% of the data. It is a measure of variability and helps in understanding the spread of the data.
2. How can I visualize the IQR?
One of the best ways to visualize the IQR is through box plots, where the box represents the IQR, covering the middle 50% of data, and the whiskers show the range of the rest of the data, allowing for quick assessment of data spread and outlier detection.
3. Why is calculating IQR important?
Calculating the IQR is important for evaluating data variability and identifying outliers, which can distort analytics and interpretations in research and business scenarios. It enables a clearer understanding of the core of the dataset.
4. Can I calculate IQR without software?
Yes, the IQR can be calculated by hand by following a systematic process: sort the data, find Q1, find Q3, and then apply the IQR formula. Even though it may be more time-consuming than using statistical tools, it is entirely feasible.
5. What role does IQR play in box plots?
In box plots, the IQR is visually represented by the box itself, where it indicates the variability of the central 50% of the data. It serves as a tool for understanding the data's spread and the possibility of outliers.
6. What are some real-world applications of IQR?
The IQR finds applications across various fields such as education for analyzing student performance data, finance for assessment of stock market volatility, and medicine for evaluating clinical trial data. It provides a means to gauge the consistency of outcomes in these areas.
7. What do high or low IQR values indicate?
A high IQR suggests a wide spread in the central data points, indicating significant variability, while a low IQR reflects that the middle 50% of the data is clustered closely together, suggesting less variability among those data points.
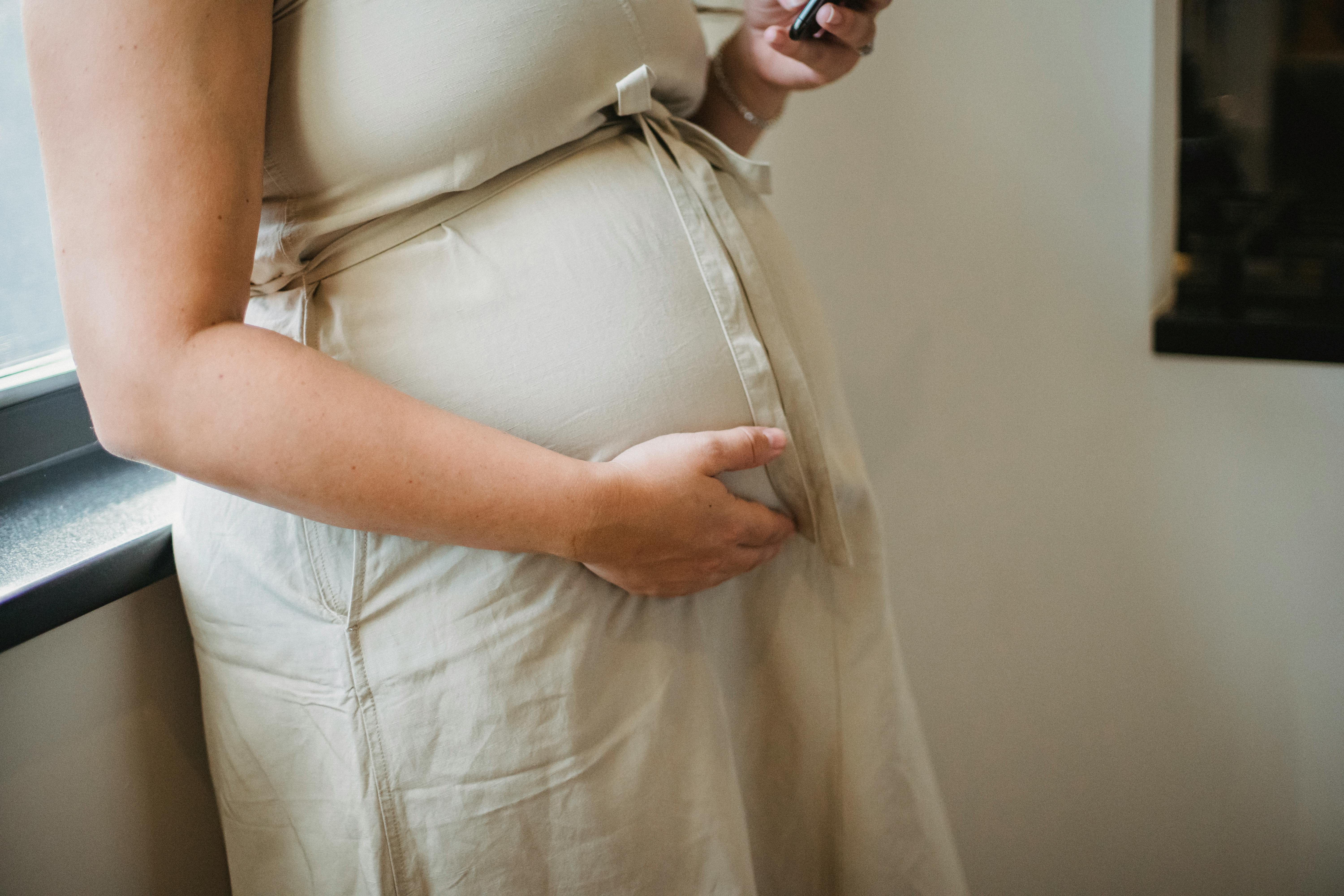
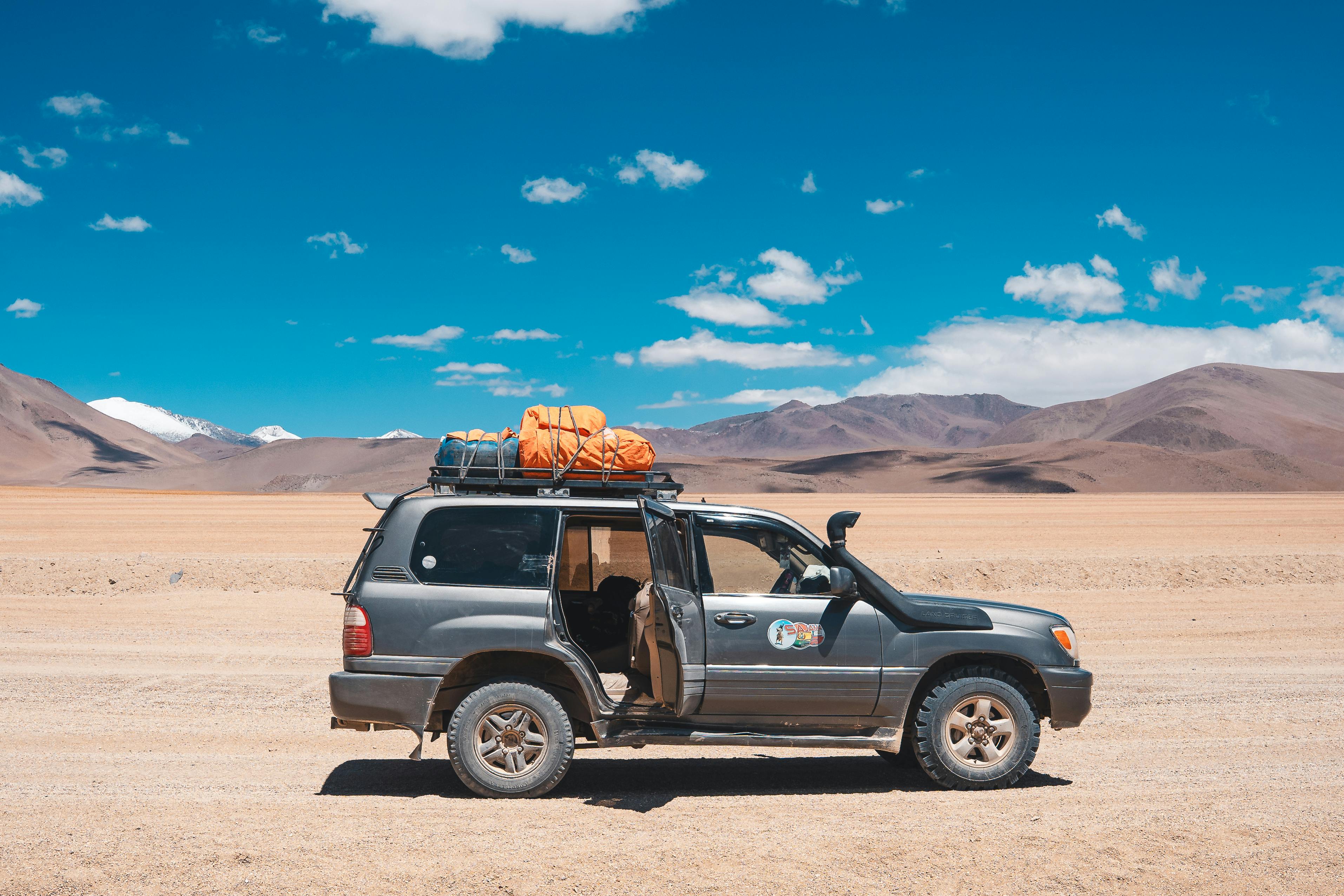