Smart Ways to Determine the Confidence Interval for Your Analysis in 2025
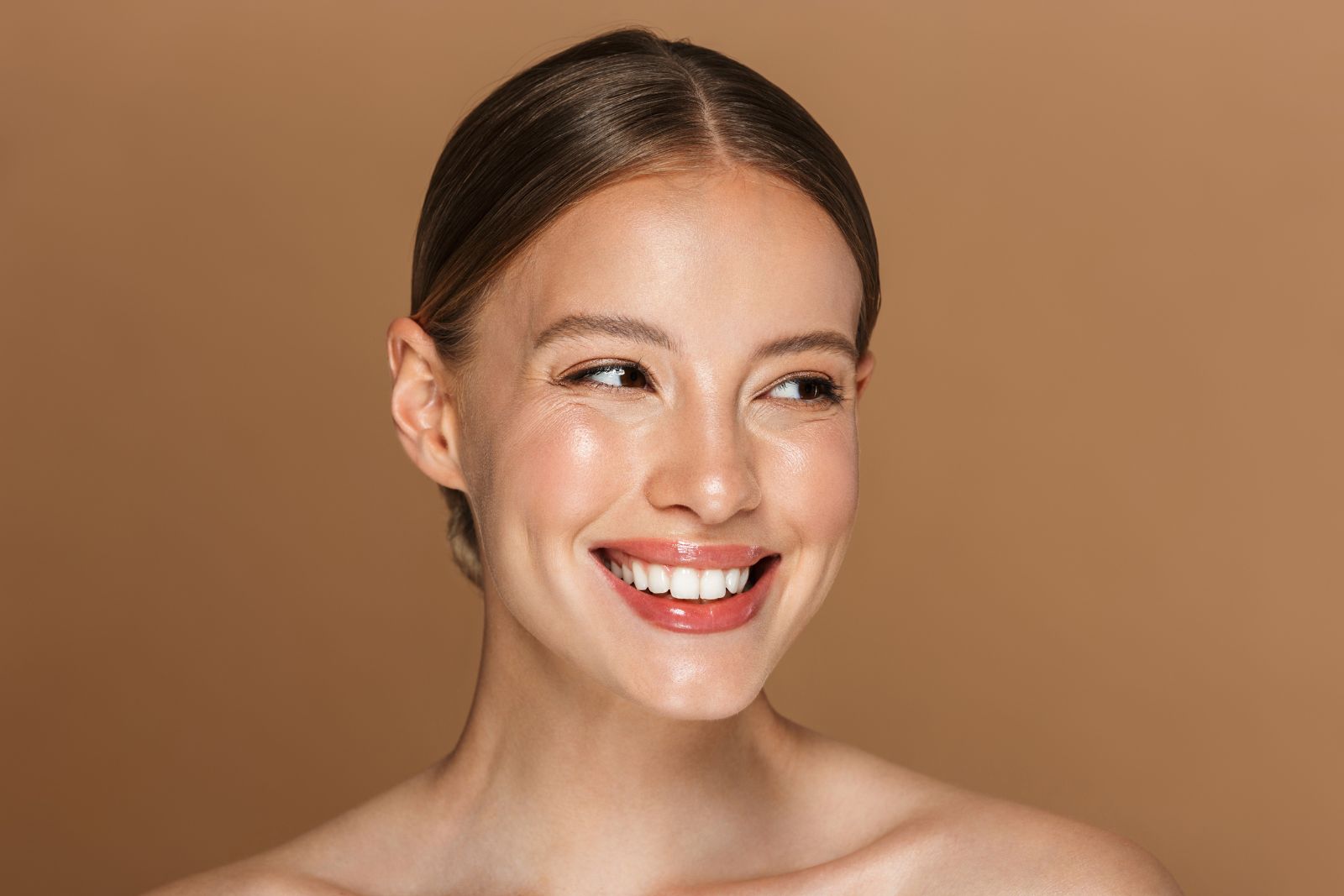
Effective Ways to Find the Confidence Interval You Need in 2025
Understanding Confidence Intervals
A **confidence interval** is a key concept in **inferential statistics**, serving to estimate the range within which a population parameter is likely to occur based on a sample statistic. Determining an appropriate confidence interval involves understanding the **confidence level** you wish to achieve—common levels include 90%, 95%, and 99%. The **margin of error** reflects how much variation can be tolerated. A broader interval indicates more uncertainty but also greater confidence that you’ve captured the true population parameter. Confidence intervals not only aid in making predictions but also form the cornerstone of **statistical analysis** in decision-making.
The Confidence Interval Formula
The **confidence interval formula** typically looks like this: CI = sample mean ± (critical value × standard deviation of the sample). The critical value can be derived from the **standard normal distribution** or the **t-distribution**, depending on sample size and whether the population standard deviation is known. The **sample mean** serves as your point estimate, while the standard deviation provides a measure of variability. It's important to consider that as the sample size increases, the confidence interval narrows due to reduced uncertainty about the population parameter.
Importance of Understanding the Confidence Level
The choice of confidence level greatly impacts your results. For instance, opting for a **95% confidence level** means you can expect that 95 out of 100 samples would generate intervals that include the true population parameter. However, aiming for a higher confidence level, such as 99%, will result in a wider confidence interval. Understanding this relationship is crucial in fields like **data analysis** and **research methodology**, where precise data interpretation can influence significant outcomes in studies.
Types of Confidence Intervals
<pThere are various types of **confidence intervals** applicable in diverse situations, such as one-sample and two-sample confidence intervals. A **one-sample confidence interval** applies when assessing a single group, while a **two-sample confidence interval** compares two different groups. Additionally, in contexts like **regression analysis**, **confidence bands** help illustrate the uncertainties surrounding your predictions. By recognizing the appropriate type and its applications, analysts can enhance their **statistical inference** and make data-driven decisions more robustly.Calculating Confidence Intervals: Step-by-Step Guide
Calculating confidence intervals entails a systematic approach. Firstly, ensure that you have a reliable **sample statistic** from your data collection efforts. Next, determine the **margin of error**, which involves knowing the **z-score** or critical value applicable to your desired confidence level. Finally, apply the confidence interval formula based on your assessments. In this section, we will delve into a practical example to clarify these steps.
Practical Example: One-Sample Confidence Interval
Suppose we have a sample mean of 500, a sample standard deviation of 50, and a sample size of 30. To find the **confidential interval**, we first determine the critical value for 95% confidence, which is approximately 1.96 (using the z-distribution since our sample size is sufficient). The next step is to calculate the **margin of error** (1.96 * (50/sqrt(30))), yielding about 18. For the final step, we compute the CI as follows: CI = 500 ± 18, resulting in (482, 518). This means we're 95% confident that the true population mean lies within this interval.
Using Statistical Software for Confidence Intervals
With advancements in data analysis, software tools like R, Python, or statistical calculators have simplified the process of finding confidence intervals. These applications can automate calculations, reduce manual errors, and provide instant visualizations. All one needs is the data inputs—the software will compute the confidence interval for you, significantly enhancing efficiency in various **research statistics** and daily data analysis tasks.
Importance of Sample Size in Confidence Intervals
The accuracy of your **confidence intervals** heavily relies on **sample size determination**. A larger sample size typically produces a smaller margin of error and consequently a more precise confidence interval. It is essential to consider the trade-offs involved; while increasing the sample size can improve reliability, it may also require more resources and time. In practice, acknowledging how sample size affects the **variability** in your estimates allows for better planning in your research and ensures that conclusions drawn are grounded in robust data science.
Applications of Confidence Intervals in Research
Understanding how to properly apply confidence intervals is vital across various domains, especially in **clinical trials** and policy formulation. When testing medication efficacy, confidence intervals can indicate the reliability of points where treatment impacts are considered significant. Similarly, in **economics** and business contexts, confidence intervals can evaluate the variations within sales forecasts, risk assessments, and market analysis.
Confidence Intervals in Clinical Trials
In clinical research, confidence intervals are of utmost importance. They help validate drug efficacy outcomes by establishing the range in which the actual treatment effect may fall. When reporting results, showing confidence intervals alongside point estimates provides a more comprehensive picture of treatment effects, allowing for informed healthcare decisions. Regulations often require that such intervals be reported to help accurately present results to stakeholders.
Confidence Intervals in Business Analytics
Within business analytics, confidence intervals support decision-making related to market sizing and forecasting. For example, a company might use a confidence interval to analyze customer satisfaction scores, providing insights into whether strategies are effectively meeting company goals. This added layer of insight helps businesses manage risks and strategize based on statistically sound evidence.
Evaluating Prediction Intervals vs. Confidence Intervals
While often confused, it’s essential to distinguish that **confidence intervals** estimate the uncertainty around a sample statistic, while **prediction intervals** focus on predicting future observations. This demarcation is critical in statistical modeling where clear outcomes based on analyses might relate more to accurate predictions about future rows of data. An understanding of both intervals enhances data interpretation and usage in forecasts.
Key Takeaways
- Confidence intervals provide a range for estimating population parameters.
- The critical value and margin of error are crucial for calculations.
- Understanding sample size impacts is key to accurate estimations.
- Applications extend across clinical trials and business analytics.
- A clear distinction between confidence and prediction intervals enriches analysis.
FAQ
1. How do I interpret a confidence interval?
Interpreting a confidence interval involves recognizing that it represents the range where the true population parameter will likely fall based on a given sample. For instance, a 95% confidence interval implies that if you were to repeat the sample many times, approximately 95% of those generated intervals would include the true population parameter.
2. What is the critical value in finding confidence intervals?
The critical value is a factor used to compute the width of the confidence interval. It is derived from the **z-score** or the **t-distribution** based on the desired confidence level and the standard deviation of the sample. The role of this value is to scale the standard error to reflect the degree of confidence required.
3. What differences exist between confidence intervals and prediction intervals?
While both confidence and prediction intervals reflect uncertainty, the former ties to parameter estimates, whereas prediction intervals serve to forecast an individual observation's likelihood. Understanding this difference is critical for analysts when conveying results and making predictions based on statistical models.
4. How does sample size affect confidence intervals?
A larger sample size usually leads to narrower confidence intervals because it reduces the margin of error. This is due to increased reliability in estimating the population parameter; hence more data typically offers better accuracy and precision in estimation.
5. Why are confidence intervals important in research methodology?
Confidence intervals provide researchers with critical insights into the reliability of their findings. They help quantify uncertainty, allowing for making informed decisions and recommendations based on statistically valid conclusions. The transparency they introduce enhances the credibility of analyses and findings in any research-oriented study.